Authored by: Jizelle Dumayas
Art by: Michelle Choi
The use of Artificial Intelligence (AI) in medical algorithms for clinical interpretations (i.e. patient diagnosis) can be a harmful practice in healthcare, specifically towards minority populations, as its reliance on data does not provide clear insight into the demographics, lifestyle, and background of diagnosed patients. Despite these concerns, it is still being used in medicine to allocate resources to patients identified with higher risk in settings with limited resources, provide accessibility to those in remote areas, and precisely identify abnormalities and provide early detection to diseases like cancer [1].
Now, what exactly is an AI algorithm? Algorithms made by AI are mathematical models that make predictions or suggestions using data collection. In medicine, they are utilized to guide medical professionals in patient diagnosis and care [2]. Insurance companies also use them to predict how to allocate healthcare resources [3]. However, according to Lucila Ohno-Machado, professor of medicine and deputy dean for biomedical informatics at Yale School of Medicine, algorithms are limited to vague and generalized data that do not represent the total population, creating biases against minorities. She says these biases "'... arise from incorrect assumptions about particular patient populations…" [2]. Bias against underrepresented groups are complications that come with the advancement of AI technology in medicine.
There is limited transparency about the algorithm's development to physicians and the public, which is why pinpointing and regulating the flaws that lead to bias requires a complex approach [4]. Another complication is that measuring racism can be subjective, which makes bias mitigation an evasive process. According to Brown Hospital Medicine, each stage of the machine's learning process is prone to racial bias, including– the harvesting of raw data, the extraction, organization, and development of the model, and the implementation of AI results [5].
Since these stages overlook key patient characteristics, like lifestyle and socioeconomic background, the resulting algorithms reinforce an implicitly biased system favoring White individuals [6]. This is why algorithms in healthcare can be referred to as "race-adjusted," as physicians use them to formulate individualized risk assessments that "... may redirect more attention or resources to White patients than to members of racial and ethnic minorities" [7].
While AI algorithms can be flawed, they also offer benefits, such as the early detection of cancer, access to healthcare in remote areas, and efficient resource allocation where resources are scarce. The National Cancer Institute recognizes AI's accuracy in recognizing "cancer-suspicious areas" in MRI and X-rays that less-experienced radiologists may overlook, potentially leading to earlier diagnoses and improved patient outcomes [8].
Although algorithms in medicine can improve efficiency in care, they are not inclusive to minority groups as they lack demographic data beyond the scope of medicine. They leave out factors like income and the communities patients are from, which influence access to healthcare [9]. It is crucial that we end misinterpretations to relieve healthcare disparities.
An example of a harmful assumption is the belief that Black patients are healthier in comparison to their White counterparts. This assumption was based on data that said Black patients were less likely to be on the national kidney transplant waitlist. However, the algorithm did not know that it was more difficult for Black patients to obtain a spot on the transplant waitlist [10].
Due to the lack of extrinsic information, underrepresented patients are often misdiagnosed or incorrectly deemed to require less medical attention [11]. Looking closer into the complications of these misdiagnoses, experts have discovered that many of these biased algorithms "... require racial or ethnic minorities to be considerably more ill than their White counterparts to receive the same diagnosis, treatment, or resources" [2].
Amidst ongoing regulations and oversight of inequitable algorithms mandated by federal agencies, nonprofit organizations and experts who actively incorporate these tools into medical practice–such as physicians–must be highly informed and prepared for possible misleading information produced by algorithms. Healthcare providers must recognize which populations are most vulnerable to misrepresentation and understand the lifestyle factors that may influence these patients' health outcomes [12]. It is within the interest of all healthcare providers and administrators that care be offered equally for all, and one of the first steps towards meeting that goal includes improving misinformed algorithms that continue to perpetuate institutionalized inequality.
Ways to mitigate biases created by medical algorithms include a set of five stages: (1) Identifying the issue that the algorithm is made to address; (2) Manually selecting and managing the data so that it is diverse and representative of the target population; (3) Validating the algorithm; (4) Deploying the algorithm; and (5) Continuously evaluating the performance and results of the algorithm. These steps apply to both the professionals involved in algorithm development (e.g., AI developers, researchers, and data scientists) as well as healthcare providers (e.g., physicians) who apply them in practice.
While AI algorithms can enhance diagnostic efficiency, they magnify disparities among minority populations when they fail to account for diverse demographics of these groups. To resolve these issues, healthcare providers must adopt more inclusive data practices to provide equitable care for all patients [2].
Works Cited
Ellis, L. D. (2024, August 30). The benefits of the latest AI technologies for patients and
clinicians. The Benefits of the Latest AI Technologies for Patients and Clinicians | HMS Postgraduate Education. https://postgraduateeducation.hms.harvard.edu.
Backman, I. (2023, December 21). Eliminating Racial Bias in Health Care AI: Expert Panel
Offers Guidelines. Yale School of Medicine. https://medicine.yale.edu.
How Health Care Algorithms and AI Can Help and Harm. Johns Hopkins Bloomberg School of Public Health. (2023, May 2). https://publichealth.jhu.edu.
Grant, C. (2022, October 3). Algorithms Are Making Decisions About Health Care, Which May Only Worsen Medical Racism: ACLU. American Civil Liberties Union. https://www.aclu.org.
Jindal, A. (2022). Misguided Artificial Intelligence: How Racial Bias Is Built Into Clinical
Models. Journal of Brown Hospital Medicine, 2(1). https://doi.org/10.56305/001c.38021
Mahmood, I., & Pettinato, M. (2021b). Impact of intrinsic and extrinsic factors on the
pharmacokinetics of peptides: When is the assessment of certain factors warranted? Antibodies, 11(1), 1. https://doi.org/10.3390/antib11010001
Vyas, D. A., Eisenstein, L. G., & Jones, D. S. (2020). Hidden in plain sight — reconsidering the use of race correction in clinical algorithms. New England Journal of Medicine, 383(9), 874–882. https://doi.org/10.1056/nejmms2004740
Jaber, N. (2022, March 22). Can Artificial Intelligence Help See Cancer in New, and Better, Ways?. Can Artificial Intelligence Help See Cancer in New, and Better, Ways? - NCI. https://www.cancer.gov.
Bajwa, J., Munir, U., Nori, A., & Williams, B. (2021). Artificial Intelligence in healthcare:
Transforming the practice of medicine. Future Healthcare Journal, 8(2). https://doi.org/10.7861/fhj.2021-0095
Friend or foe: A closer look at the role of health care algorithms in racial and ethnic
disparities. The role of health care algorithms in racial bias, inequity. (2024, March 25). https://www.pennmedicine.org.
Mahmood, I., & Pettinato, M. (2021b). Impact of intrinsic and extrinsic factors on the
pharmacokinetics of peptides: When is the assessment of certain factors warranted? Antibodies, 11(1), 1. https://doi.org/10.3390/antib11010001
Cary, M. P., Zink, A., Wei, S., Olson, A., Yan, M., Senior, R., Bessias, S., Gadhoumi, K., Jean-Pierre, G., Wang, D., Ledbetter, L. S., Economou-Zavlanos, N. J., Obermeyer, Z., & Pencina, M. J. (2023). Mitigating racial and ethnic bias and advancing health equity in Clinical Algorithms: A scoping review. Health Affairs, 42(10), 1359–1368. https://doi.org/10.1377/hlthaff.2023.00553
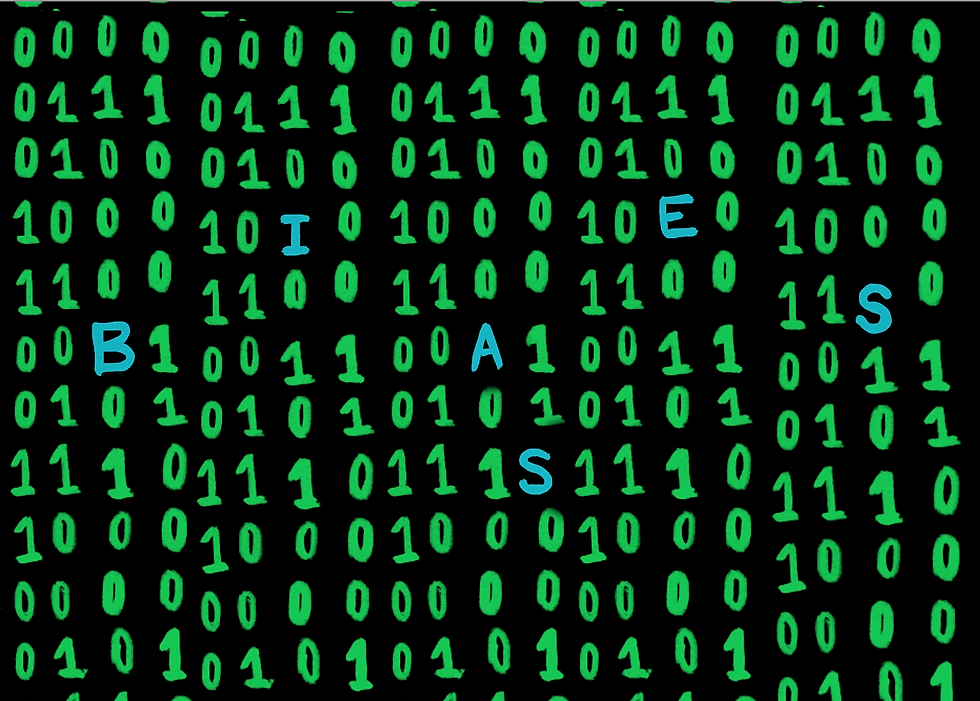
Comentarios